We overlaid a 200 meter grid on Prospect Park, ignoring features and topographic variation. Each point was identified by its latitude and longitude and an idealized snaking path that connected them. Our intention was walk to each point, collect data along the way, and capture additional data at each point.
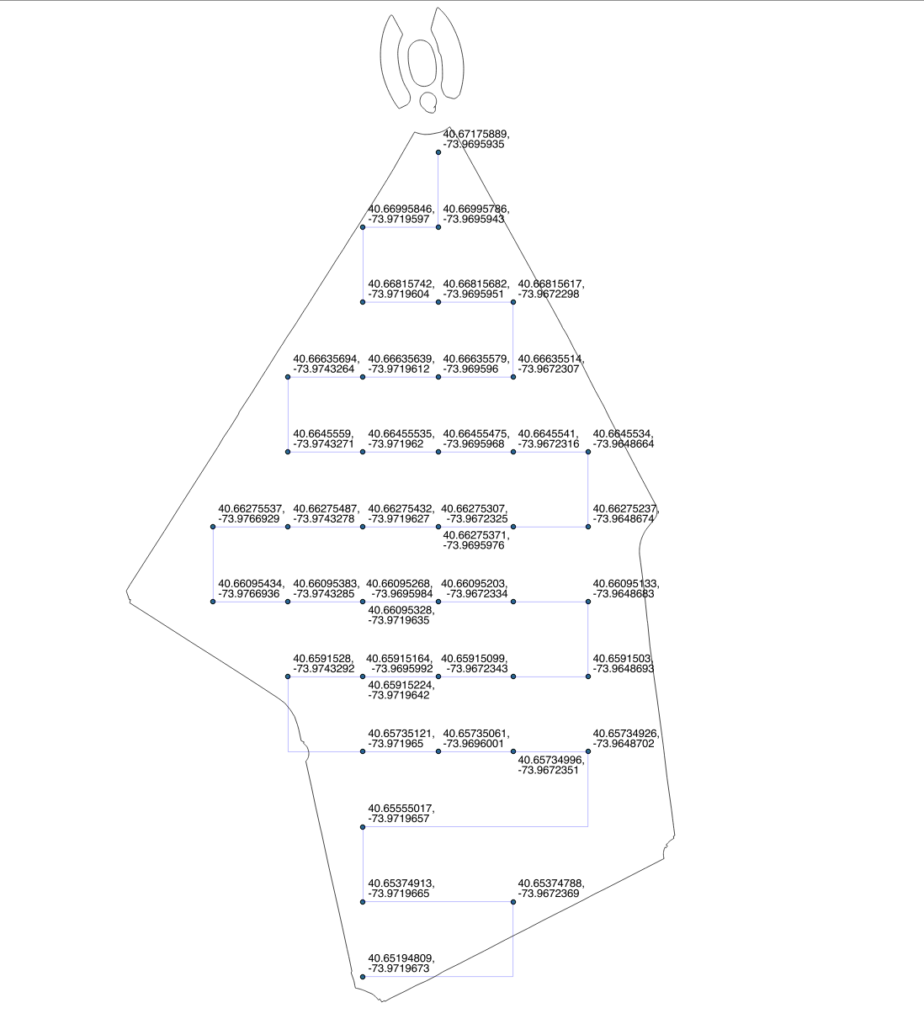
Prior to starting the walk, directions were generated using Google Maps between sets of 8 points to get a sense of time and distance. Google Maps was used as it has more specific path data than alternative platforms such as Apple Maps.
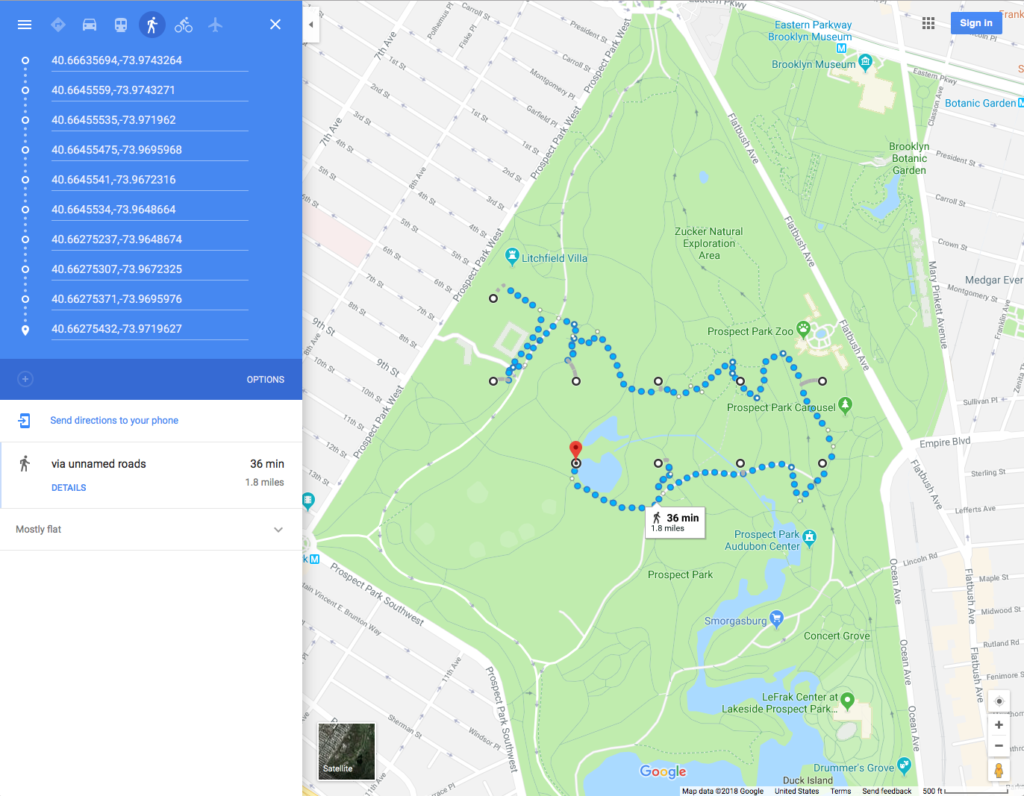
Once walking, these directions were abandoned in favor of entering each coordinate pair individually, point-by point. Occasionally, this method suffered from human error — entering the wrong point — evident by a deviation in the recorded path.
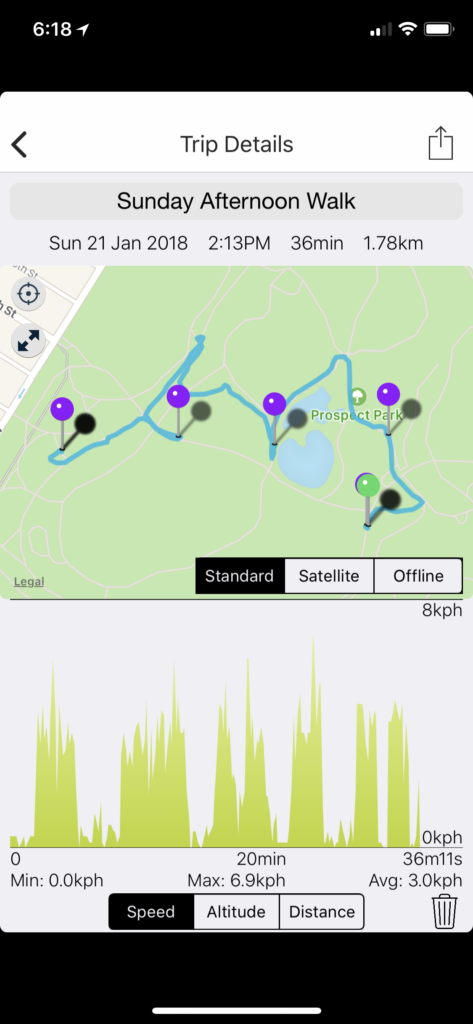
While walking, GPS position, accuracy, and altitude were captured using a Bad Elf GPS Logger. The track was automatically recorded at 12 second intervals, and when an intended grid point was reached, the position was marked as a point-of-interest. Additionally, three photos were taken of the GPS logger and two screenshots of its corresponding phone app to provide back up data. A photo of the sky, while facing north, was also taken at each point, to describe the conditions affecting the accuracy of the GPS positioning.
The impression was that data collection would be straightforward–we had coordinates on a grid and directions to each point. Using Apple and Google Maps, we assumed that if we entered exact coordinates, we would be routed to exact coordinates. As it turned out, mapping services are based on approximations. You don’t arrive at your destination so much as you arrive in its general proximity, with the presumption that you’ll be able to find your way from there. This illustrates the scale in which these services operate — three to five meters — and a remnant of human cognition left in the navigation process. These services foster an illusion of getting you where you need to go, but they only take you part of the way, and only on paths known to the services.
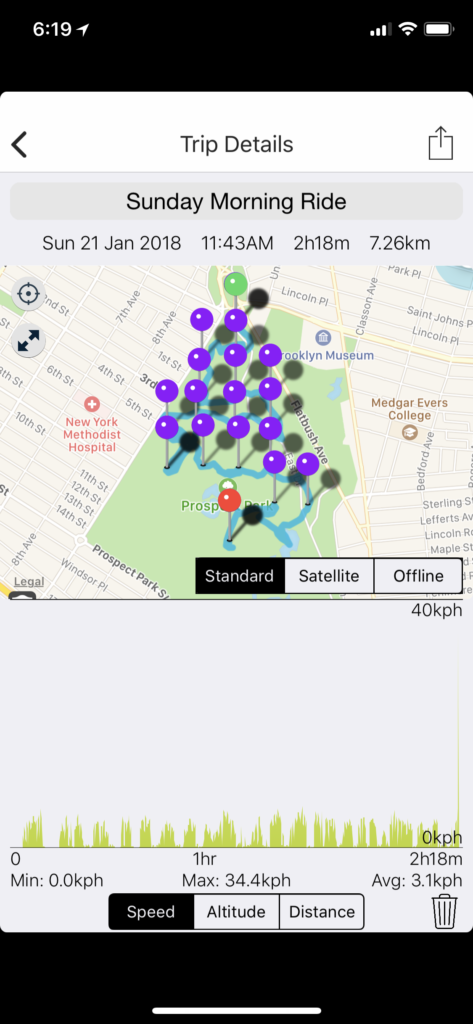